Generative AI has emerged as a potentially transformative force in the healthcare industry, already showing use cases that can revolutionize patient care, diagnosis, and treatment. Its potential to enhance clinical decision-making, streamline operations, and improve patient outcomes cannot be understated. I’m already hearing clients ask for help with governance and security.
However, as generative AI continues to gain traction, executives and decision-makers in the healthcare sector must recognize and address the ethical implications that go hand-in-hand with its adoption.
Ethical considerations such as transparency, accountability, fairness, and privacy must be at the forefront of AI initiatives, ensuring that patient well-being and trust are upheld throughout the AI implementation process. By acknowledging and proactively addressing these ethical implications, healthcare organizations can navigate the complex landscape of generative AI and harness its transformative power while upholding the highest standards of ethical conduct.
To hear practitioner and platform insights on how solutions such as ChatGPT will impact the future of work, customer experience, data strategy, and cybersecurity, make sure to register for your on-demand pass to Acceleration Economy’s Generative AI Digital Summit.
Key Ethical Considerations in Generative AI for Healthcare
Transparency, explainability, bias, and fairness are crucial aspects to consider when dealing with generative AI algorithms in healthcare. Executives should prioritize understanding and communicating how generative AI systems generate their outputs. This transparency enables healthcare professionals to comprehend decision-making and fosters stakeholder trust. Explainability also helps identify and address any biases or errors that may emerge from generative AI algorithms, ensuring fairness and accountability in healthcare applications that utilize generative models.
To understand the risk of bias in generative AI algorithms, consider a case where a facial recognition algorithm, part of a generative AI application, exhibits racial bias by misidentifying individuals with darker skin tones. This bias can lead to inequitable healthcare outcomes and exacerbate disparities in treatment.
To ensure fairness, imagine a generative AI algorithm recommending treatment plans in a clinical decision support system. If the algorithm predominantly recommends costly treatments without considering patient-specific factors like financial constraints or individual preferences, it may result in unfair treatment recommendations and compromised patient autonomy.
When we mention diverse and representative datasets, think of a generative AI algorithm being trained on electronic health records (EHRs) from a specific demographic, resulting in a lack of racial or socioeconomic diversity. This narrow dataset can lead to biased predictions and limited ability to generalize the algorithm’s outcomes for a broader population.
Explainability becomes crucial when considering any generative AI algorithm that assists radiologists in diagnosing conditions like lung cancer from medical imaging scans. It is vital for the algorithm to provide interpretable explanations for its predictions, enabling radiologists to understand how the algorithm arrived at a specific diagnosis. This helps build trust and confidence in the AI system and empowers clinicians to make informed decisions.
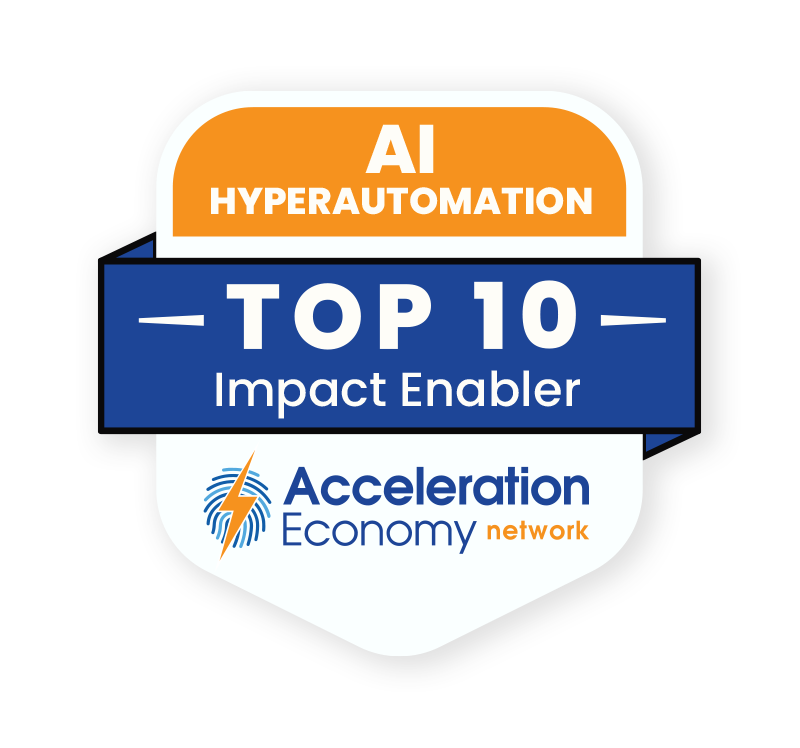
Which companies are the most important vendors in AI and hyperautomation? Check out the Acceleration Economy AI/Hyperautomation Top 10 Shortlist.
Real World Transparency and Ethics of Generative AI
A great place to begin to understand how vendors plan to tackle ethics and bias issues is to look at some of the early work Microsoft and others are doing. For example, the Microsoft Guidance repository on GitHub offers valuable guidance on mitigating biases and promoting fairness in generative AI applications.
Executives can utilize the resources provided to identify potential biases within generative AI algorithms and datasets, implement bias-mitigation techniques, and continuously evaluate and monitor for fairness. By following the repository’s recommendations, healthcare organizations can take proactive steps to ensure that generative AI algorithms provide fair and equitable healthcare outcomes for all patients.
Incorporating diverse and representative datasets, leveraging algorithmic fairness techniques, and conducting regular audits are key elements in promoting fairness and reducing biases in generative AI-driven healthcare applications.
Oracle’s recently published “Guide to Ethical Considerations in AI Development and Deployment” provides insights into addressing ethical considerations in the adoption of AI in healthcare. It emphasizes the importance of transparency, fairness, accountability, and privacy in AI systems. The whitepaper offers practical guidance on implementing responsible AI practices, such as ensuring algorithm explainability, mitigating biases, and protecting patient data. It highlights the significance of ongoing monitoring, evaluation, and governance to uphold ethical standards throughout the AI lifecycle.
By leveraging these practices, executives can navigate the complexities of responsible AI adoption in healthcare, foster trust among stakeholders, and drive positive patient outcomes while upholding the highest ethical standards. Both Microsoft and Oracle are working on tools to assist in automating tasks related to ethics and checking models. The Microsoft Dashboard for Responsible AI is a great example of these tools.
I predict many other vendors will develop similar approaches and tools.
Conclusion
In the rapidly evolving landscape of generative AI adoption in healthcare, the ethical considerations surrounding transparency, fairness, and privacy are crucial for technical executives to address. Fortunately, there’s plenty of help for this, as evidenced by the examples from Microsoft and Oracle above. By leveraging these resources, executives can navigate the complexities of responsible generative AI adoption and ensure the ethical implementation of AI in healthcare.
Want more tech insights for the top execs? Visit the Leadership channel: