The newly released cloud version of RapidMiner’s data science platform adds important new functions aimed at driving up the impact of artificial intelligence (AI) technology across an organization by facilitating the deployment of more AI models and positioning more users to take advantage of its benefits.
Company leaders Peter Lee (CEO) and Ingo Mierswa (Founder and Chief Technology Officer) noted that AI’s potential to drive impact and digital transformation within an organization can be limited if there’s insufficient usage of AI models, due to a lack of skills or knowledge.
The approach they’re taking is to make AI easier to use and deploy, expand its use, and equip people to fully leverage it whether they have a data science background or not.
According to the company, AI today is impeded by three factors:
- Inability to hire enough data scientists
- Organizations lack data science familiarity and comfort level — it’s a “black box”
- AI requires IT flexibility and capacity that may or may not be available
The result: Not enough of the AI models that get built make it into production, so companies don’t get (or perceive) adequate value from their investment in AI technology.
Lee summed up the challenges with one comment that particularly resonated around the people requirements for success at digital transformation: “Digital transformation is more so about people transformation than tooling transformation.”
RapidMiner’s latest release and directions address the problem in multiple ways:
Offering a fully cloud-based version (as previously noted) to ensure maximum accessibility and alignment with the ubiquitous IT architecture. Interestingly, that was not a major focus in this week’s presentation, but it’s obviously of critical importance.
Expanding the number of people who can capitalize on the technology, including through “fully automated AI” that does not require ML expertise or dealing with IT infrastructure. Automated AI understands underlying patterns in your data, can define goals, run analyses, and start computations.
If a company has a business expert who is data-savvy but doesn’t code, it can tell RapidMiner what it would like to predict and the system will create a model, generate insights, and predict the future based on those models. “You can be proactive, make changes and make improvements; it’s a way to simply generate insights,” Mierswa says.
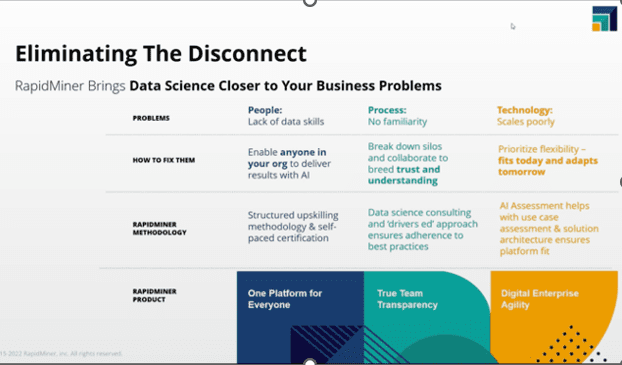
Automated AI is built to overcome the lack of familiarity problem that can plague AI. RapidMiner expresses this concept as “bringing data science closer to your business problems.”
Fully automated is one of three authoring “modalities” that RapidMiner offers. The others are “notebooks” (for experts) and workflow designer (which allows experts to inspect data lineage, types of models, and how models operate downstream).
“Our platform is designed to support all people in an organization, from novices to experts,” Lee said
Lower the barriers to deployment. Execs noted the challenges that organizations face in deploying AI models into production. A new AI assessment methodology helps IT and the business prioritize use cases. The company also will devise the optimal technology architecture. But the core goal is to address the scalability issue of models not getting into production. “You don’t need to deal with any IT infrastructure,” Mierswa says.
Building trust in AI that leads to broader usage. Lee and Mierswa explained the importance of sharing insights derived from AI models, and demonstrated apps that can be built on the fly, using a drag-and-drop methodology, pulling in data from systems and models so that insights can be shared broadly. It’s an accepted principle of analytics that transparency breeds confidence and perceived value, so the apps functionality in RapidMiner addresses that point directly – and should, in fact, lead to broader usage.
Here’s one example of an app screen designed to address the trust/confidence consideration:
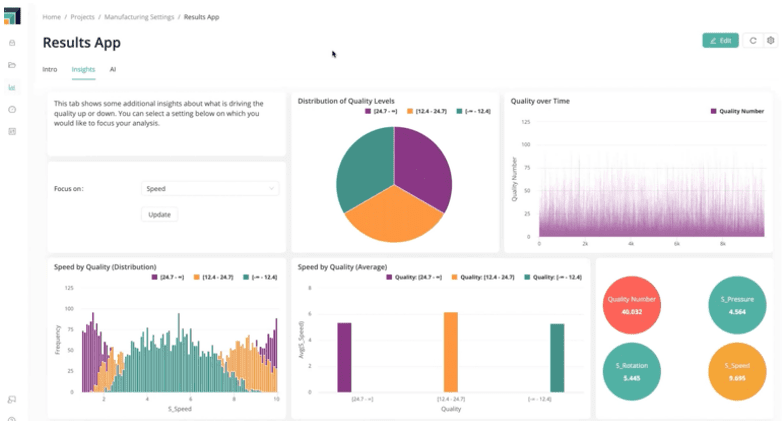
Training for all levels of users through an AI academy program that leaders compared to driver ed: sitting next to the client or user, focused on helping them build skills and confidence as they adopt best practices in AI. The company is also building curriculum for repeatable use cases.
“If we do our job correctly, we’ll see clients have tremendous gains in their digital journey, they’re able to maximize business outcomes from multiple use cases across functions, and they’re going deep within any specific function,” Lee says. “We’re focused on building expertise and self-confidence to implement a data-driven culture.”
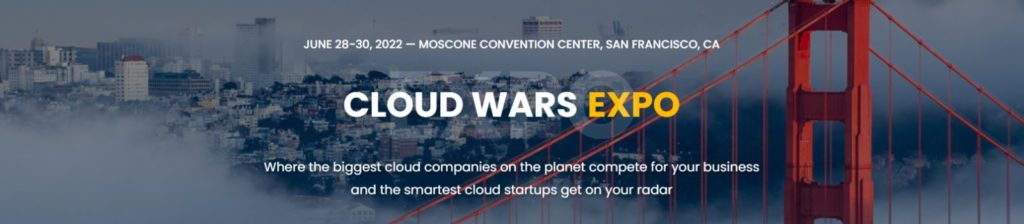