These days, there’s a lot of money in AI. Maybe you caught wind of some of the recent funding and M&A headlines:
- Microsoft pitching billions into OpenAI, raising OpenAI to a $40B valuation
- Salesforce investing half a billion into Anthropic
- Runway raising another $141M
- Snowflake acquiring Neeva
Perhaps you also heard that Databricks announced a $1.3 billion acquisition of MosaicML. In this analysis, I expand on the significance of this acquisition and my previous commentary, with the context of the other acquisitions mentioned above.
Databricks Differentiators
Databricks is one of the Acceleration Economy Top 10 companies in the Data Modernization category and a pioneer in data management. Based in California, the company was founded in 2013 and was last valued at $38 billion.
It provides a unique offering that combines the data warehouse approach — a data management architecture designed in the 80s for structured data that is easy to query — with the data lakehouse approach. A data lakehouse is a more modern architecture that is designed for unstructured data. Databricks’ platform combines the ease of querying offered by data warehouse systems with the flexibility of data lakehouses.
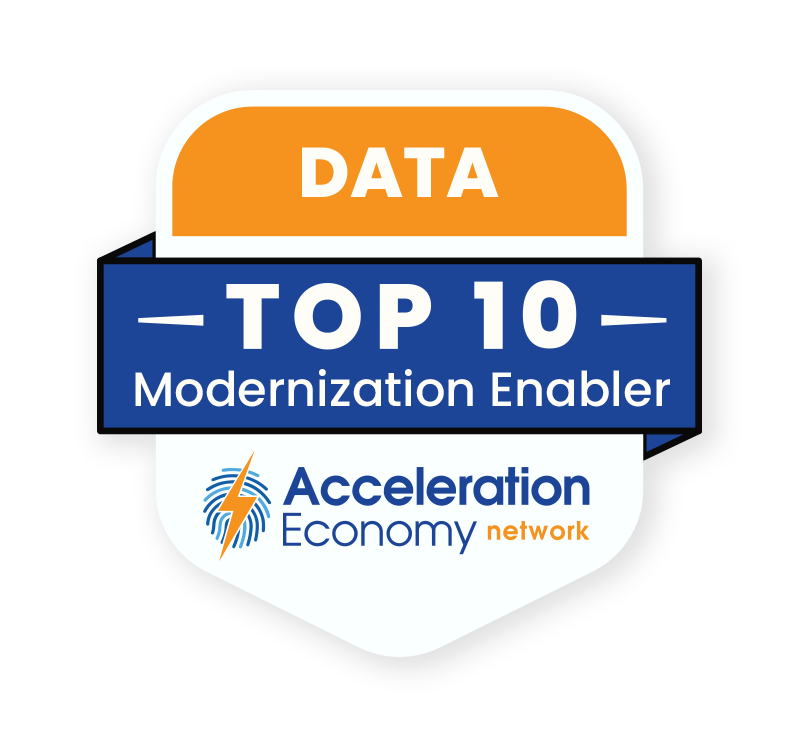
Which companies are the most important vendors in data? Check out the Acceleration Economy Data Modernization Top 10 Shortlist.
Who is MosaicML?
As mentioned, MosaicML is a relatively young company based in San Francisco. $1.3 billion is a hefty price tag given the size and age of MosaicML, a company founded in 2021 with under 100 employees listed on LinkedIn. Not to mention its last investor round valuation was $222 million. Part of the reason for this huge price tag may be that the entire MosaicML team — many of whom are highly sought-after AI engineers — will be joining Databricks.
MosaicML’s main objective is to let any organization build custom ML models using their own data. To reach this goal, the company built a platform that lets organizations train their own models and deploy them. Customers are offered two options: custom pre-training with their own data or fine-tuning of models that MosaicML has pre-trained. It is worth noting that MosaicML open-sourced these pre-trained models and it never stores customers’ data for future training.
While the foundation models themselves are open-sourced, the process and platform for implementing them is MosaicML’s product. The company offers its MosaicML Training package, which allows customers to navigate the entire training process while protecting internal data and controlling the final model weights. Pricing is per GPU minute. It also offers direct access to the foundation models via an API endpoint which is priced per 1,000 tokens. Enterprises can choose to deploy models via MosaicML-managed compute, another cloud provider, or on-premises.
The Importance of the Databricks MosaicML Collaboration
With this acquisition, the MosaicML platform will join the Databricks Lakehouse Platform, adding enterprise-friendly generative AI tooling to Databricks’ existing multi-cloud offerings. This adds value to both companies’ existing customers.
As mentioned, MosaicML open-sourced its foundational models and chooses not to store customer data. This stems from its belief in a future that is not dominated by a single model or a single company. AI is too powerful of a technology to be controlled by a monopoly, and more value is unlocked if everyone has access to the models.
But there’s also tremendous value to the buyer. MosaicML’s approach lets customers escape vendor lock-in, a common concern when working with proprietary foundational model providers including Meta, Google, OpenAI, and others. It also reduces the risk profile of working with a provider by giving the customer full control of the training process and by choosing not to store customer data, a concern that cropped up in the recent case of Samsung employees leaking sensitive data to ChatGPT, for example. The flexibility of MosaicML’s platform also gives customers more control over compliance and costs.
Final Thoughts
Overall, this strategic investment adds value to MosaicML, Databricks, and the customers of both companies. It’s like two Lego bricks that snap together — you have a company pioneering data management and you have a company that enables customers to build models on top of their own data. Merging the capabilities of these two companies reduces the friction and time-to-value for customers implementing bespoke models.