Drop a prompt request into a generative artificial intelligence (AI) engine and out pops the meat of a business plan, a detailed educational article, or a ready-to-use email campaign sequence. Pretty well-written content filled with rich information in a usable structure. Quick. Done.
That’s the upside of generative AI.
On the other hand, examples are emerging of generative AI gone wrong. A lawyer makes a case using info from an OpenAI engine based on erroneous information. A product leader uses outdated statistics to make claims as part of an industry product launch. And a generative AI-produced article in an industry magazine publishes non-existent expert quotes. These are just a few I’ve heard recently.
Generative AI is different from other kinds of AI. First, it’s accessible to any business or tech user, not just data scientists and developers. Second, its outputs are mesmerizing to the user. Large language models (LLMs) respond to user prompts with natural language outputs that convincingly mimic human language.
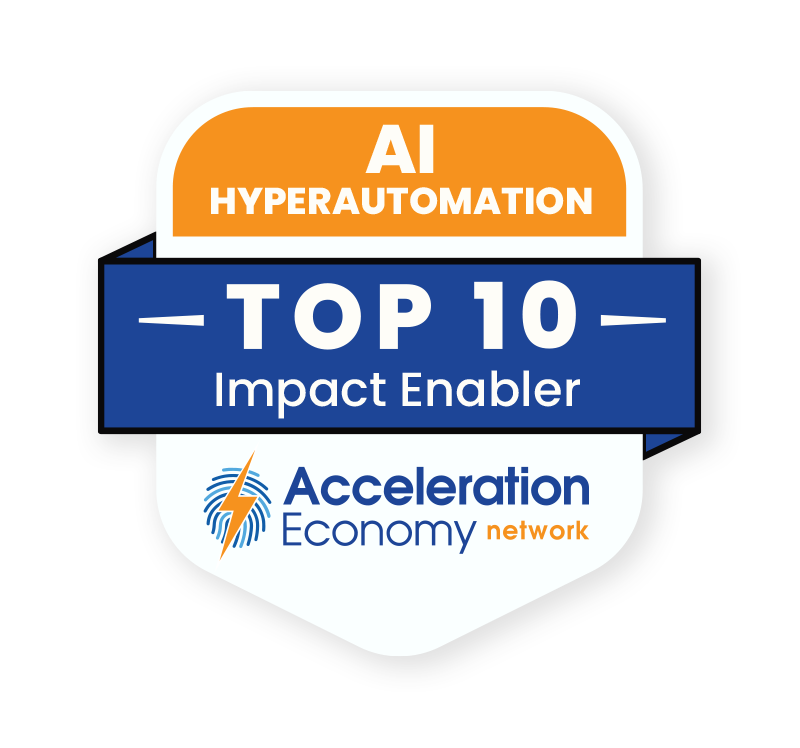
Which companies are the most important vendors in AI and hyperautomation? Check out the Acceleration Economy AI/Hyperautomation Top 10 Shortlist.
Many eager professionals in your organization may not know how generative AI works or how much confidence to have in its outputs. The speed of AI innovation is outpacing companies’ ability to understand, let alone manage, the risk. This is where leaders can take control. Generative AI can be used more confidently when you understand and mitigate risk from the outset.
Which companies are the most important vendors in AI and Hyperautomation? Click here to see the Acceleration Economy Top 10 AI/Hyperautomation Short List, as selected by our expert team of practitioner-analysts
What Can Go Wrong?
I usually like to start with the positive. But as C-suite leaders focus on enabling people, it is essential to understand the inherent risks of generative AI, especially at this early stage of use by mainstream workers. Here are several critical dangers to understand about generative AI:
- AI that uses natural language applications can hallucinate or produce false information (however convincing the outputs may seem).
- Content produced by AI can infringe on copyright law or intellectual property rights, opening your organization to litigation.
- Information that AI models produce can be outdated and may no longer be valid.
- AI models used to design and train data can rely on biased datasets rather than diverse sources.
- AI can impersonate people or produce digital artifacts that appear to have the same fidelity as those created by humans, crushing brand trust.
- Your employees can unknowingly share personal or company information that becomes available in public datasets.
How to Navigate Generative AI’s Risks
Once you understand the risks, there are measures that organizations can take to manage them. They include:
- Develop a cross-functional team to set company guidelines and policies. Finance, data science, security, software development, marketing, sales, and business operations are important roles to have represented on a team that defines and implements company guidelines for generative AI use. Ideally, a senior executive will lead this group to ensure support from C-level leaders and the board of directors. One company I work with calls this group the “Gen AI Confidence Team.”
- Establish guidelines and protocols while working towards a generative AI governance policy. Get the fundamentals in place, and communicate about AI’s practical applications and inherent risks. A cross-functional tiger team can publish clear guidelines and practices for using generative AI. The procedures include detailing appropriate use cases and setting limits on the types of content that can be generated. These guidelines must quickly merge into the company’s broader data and AI governance policy.
- Educate leaders and empower your employees with continuous learning: The world of AI is still new, and many employees are still learning and experimenting. That’s why it’s important to educate stakeholders about the capabilities and limitations of generative AI. Proactive education helps you communicate how generative AI works, what it is designed to do, and its pitfalls and limitations. The result is empowerment of your staff to apply their knowledge and experience to evaluate the outputs of generative AI models critically.
- Ensure responsible design and training of AI systems and applications: Bias is a significant challenge with generative AI systems because models and tools are only as good as the data sets they are based on, and how diverse and representative those data sets are. This is true for both the AI systems you develop and the mainstream applications used by your team. Training and regularly evaluating the quality and accuracy of the generated output from your models and procedures is essential. While difficult because of the explosion of widely available generative AI-based tools, companies are establishing lists of approved applications for specific use cases.
Empowered Employees + Risk Mitigation = Innovation
You have seen this scenario before with other technologies. Generative AI is a prime example of new tech gaining a groundswell of adoption from business and tech users, as opposed to a technology championed by your IT department.
Its powerful, accessible capabilities can make your team more productive and impactful. What’s different is the breakneck speed of adoption, matched only by the number of new, and possibly unknown, applications that may be in use by your organization. It’s time to go into proactive mode to develop a generative AI risk plan to protect your company and empower your people.
Looking for real-world insights into artificial intelligence and hyperautomation? Subscribe to the AI and Hyperautomation channel: