Enterprise AI has a thorn in its side: bias.
To explain what bias means in the context of enterprise artificial intelligence, consider the story of AMZN.O: Amazon’s automated recruiting tool favored male candidates over female candidates because the data used to train it consisted mostly of men. This quickly makes it obvious how AI bias can damage your firm’s reputation, not to mention its business performance. But I would argue there’s another reason why tackling bias is becoming a top priority for firms adopting AI: trust.
In his phenomenal sequel “The Innovator’s Solution: Creating and Sustaining Successful Growth,” Clayton Christensen, author of the “The Innovator’s Dilemma”, points out that markets tend to judge emerging technologies by their performance above all else. This is why IBM, which had the budget to build the biggest and most powerful computers, dominated the mainframe market in the mid-20th century, Christensen explains in his book. However, as technology improves, the benchmarks change. When personal computers started hitting the market, performance wasn’t the main concern. Rather, companies began to focus on cost, reliability, speed, and size.
A similar scenario is unfolding with artificial intelligence. In some cases, the emphasis on performance is now being surpassed by ease of use, cost, and trust. As we offload an increasing number of tasks to AI, including personal and sensitive tasks, trust becomes top of mind for customers and a benchmark for scrutinizing the increasingly long list of AI suppliers and AI-powered organizations. Building and maintaining trust requires eliminating bias. To help you achieve this, I want to lay out two different types of bias — intentional and unintentional — so that you can better identify them and avoid them.
Intentional/Explicit Bias in AI
Intentional bias — also called explicit bias — refers to bias that is knowingly being expressed. You can build bias in machine learning models on purpose, and that’s not always a bad thing. In the case of AI-powered recruiting, for example, you might favor candidates with a minimum level of experience for the job. You can bake this into the model by selecting a training data set that includes predominantly those who meet the minimum level of experience, or labeling candidates with that experience as a good fit if you’re doing reinforcement learning.
However, bias can be introduced into AI systems for malicious purposes too. For instance, a malicious actor might purposely skew their data sets before selling them to the public to ensure that models trained on them would behave in a way that benefits the actor. Sometimes called ‘targeted bias,’ powerful AI systems built on flawed data sets have the potential to move markets, influence politics, or harm your organization. This is a cyber-attack, plain and simple. Because of this threat, your organization has to build security practices around algorithmic bias attacks.
The potential for damage from targeted bias is skyrocketing: over 100 million of the world’s knowledge workers and those in positions of power rely on ChatGPT to make decisions and come up with ideas. In other words, a single organization — OpenAI — operating a single tool — ChatGPT — has direct access to tens of millions of streams of thought.
Louis Rosenberg, a lifelong ethical technologist now running an AI company called Unanimous AI, spoke out against these risks in a recent Big Think article. He outlines the potential for tools like ChatGPT and Bing’s upgraded search engine to influence the user to believe things that may not be true. Some users have raised concerns over how Microsoft is managing this.
Whether purposeful or not, these tools are known to deliver incorrect information in a convincing way. For example, someone using the tools to assist with their taxes may believe the outputs too easily, include faulty numbers in their returns, and experience legal backlash as a result. A more dystopian example might include responses to questions about politics that imperceptibly promote certain parties and influence votes on a mass scale because the underlying data used to train the system was tampered with.
This is why AI-powered search engines have to place security and ethics at the forefront.
Unintentional/Implicit Bias in AI
Unintentional bias — also called implicit bias — is the most common form of bias in AI. It happens when data sets are imbalanced or the technical architecture of the data pipeline favors certain data points more than others, yielding skewed results.
Amazon’s automated recruiting tool mentioned earlier is a great example. The company only realized the hidden bias favoring male applicants over female ones after having deployed the system on real candidates and receiving substantial backlash from the media.
The issue arose because the company used its existing database of past applicants for software-related jobs at the company, which were mostly men. Therefore, more men were hired and the AI system came to associate males with good hires. Women, who were less represented in the training data, were hired less.
AI chat tools and search engines also suffer from unintentional bias toward what I call ‘vanilla answers.’ If you’ve been playing around with ChatGPT, you’ve probably noticed how many of the responses read like they’re voiced by a parrot, mimicking information you can find on any mediocre blog, subpar news feed, or unimaginative social media account.
This happens because the underlying model, GPT-3, was trained on text pulled from the Internet, giving it a strong bias towards the most common thoughts and opinions. When you’re searching for consensus, this is a good thing. But when you’re looking for creative inspiration for your marketing campaign, this lack of originality makes the tool pointless. Yes, ChatGPT is known to pass law and business school exams, but it doesn’t receive top grades. Why? Because it’s the equivalent of an average student who isn’t capable of pushing boundaries since its output is determined by the data on which it was trained.
Consider Top Vendors to Help Mitigate AI Bias
As a startup founder with limited resources, I’m always evaluating what can be done in-house and what can be outsourced to firms that have expertise that’s not central to our mission. One area that we outsource is building and implementing AI. This would otherwise require specialized knowledge, compute resources, and training data that we would have to source or painstakingly gather. This is where vendors come into play.
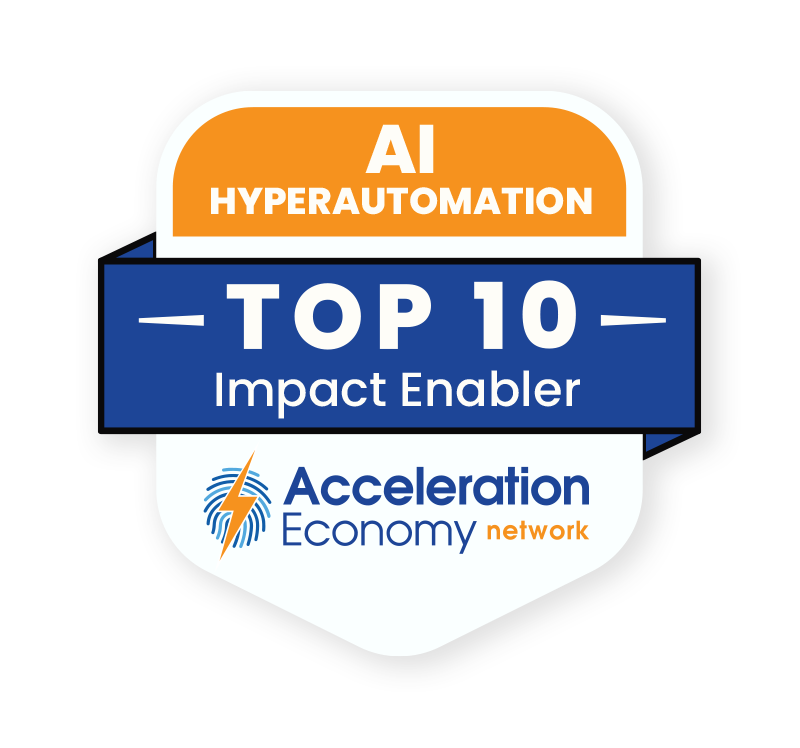
Which companies are the most important vendors in AI and hyperautomation? Check out the Acceleration Economy AI/Hyperautomation Top 10 Shortlist.
From benchmarking data set quality to choosing the right architecture to evaluating fairness and more, there is a lot to consider when it comes to preventing and mitigating bias in AI implementation. A great place to start: the vendors we chose for the Acceleration Economy AI/Hyperautomation Top 10 list based on their ability to address everything from governance frameworks to data cleaning to visualization to fairness benchmarking, and more. These firms have track records working with firms of all sizes in a range of private industries, as well as the public sector.
Conclusion
Some organizations are still taking AI implementation too lightly. While drafting a new LinkedIn post with ChatGPT is one thing, evaluating loan applications is another. Regardless of the use case, you are trusting a piece of your business to an algorithm. Furthermore, you might be banking on the trust that your customers have in your brand. Any biases that your AI holds can erode that trust which can, in turn, damage your brand. Especially if you include AI in your software or product, you must remember that fairness, trust, and safety are now at least as important as performance.
Looking for real-world insights into artificial intelligence and hyperautomation? Subscribe to the AI and Hyperautomation channel: