You can’t turn in any direction without running into a new generative AI-powered product, marketing claim, or fresh example of a company (vendor or buyer) jumping on the bandwagon. Yes, generative AI is powerful technology but it’s not yet fully understood when it comes to use cases and human impact.
Yet generative AI is in its infancy, which means we have barely scratched the surface of critical, related considerations including ethical AI and making AI explainable. This puts a huge responsibility on the shoulders of early software developers and customers who are using the technology. Why? For quite some time, I have advocated putting people first in a “People + Technology” equation, but that requires people to accept responsibility and assert control over their AI technology.
In this first of a two-part analysis, I’m going to do a deep dive to help you understand ethical AI and explainable AI, and why they’re so important. In part two, I’ll delve into why the rapid ascent of generative AI makes it urgent to address ethical AI and explainable AI in the near term.
Ethical AI – What It Is and Why It Matters
According to C3 AI, an Acceleration Economy AI/Hyperautomation Top 10 Short List company, Ethical AI — sometimes alternatively called Responsible AI — is:
“Artificial intelligence that adheres to well-defined ethical guidelines regarding fundamental values, including such things as individual rights, privacy, non-discrimination, and non-manipulation. Ethical AI places fundamental importance on ethical considerations in determining legitimate and illegitimate uses of AI. Organizations that apply ethical AI have clearly stated policies and well-defined review processes to ensure adherence to these guidelines.”
While this definition is a solid starting point, the real-world challenge that many companies have is the lack of ethical AI standards akin to the GDPR standard for handling personal data. Many companies have their own ethical AI guidelines in place, but ethical definitions and practices vary from company to company.
Myths Surrounding Ethical AI
In addition to the lack of ethical standards, the use and oversight of AI can be undermined by myths that are commonly associated with ethical AI.
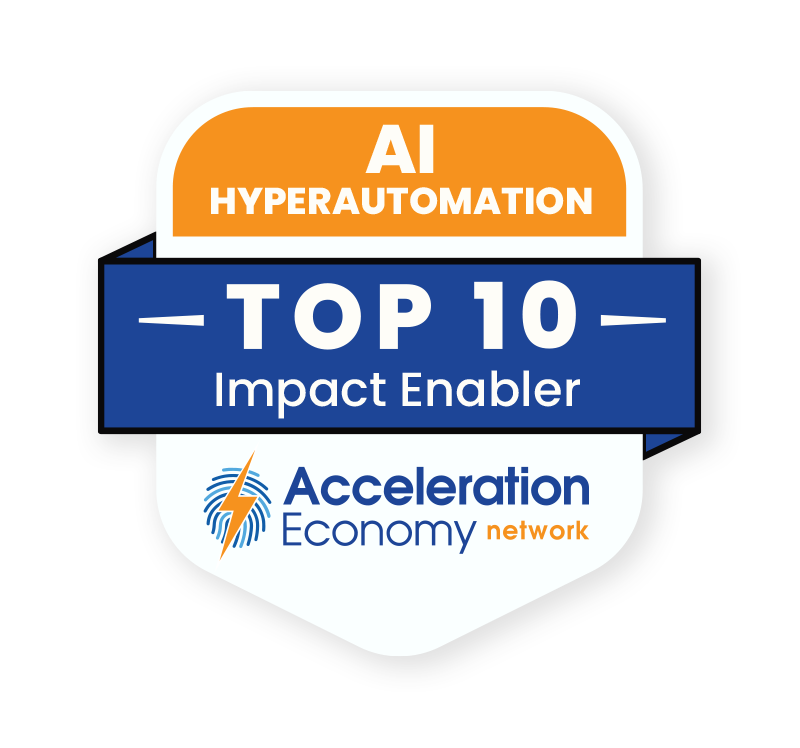
Which companies are the most important vendors in AI and hyperautomation? Check out the Acceleration Economy AI/Hyperautomation Top 10 Shortlist.
Another company on the AI/Hyperautomation Top 10 Short List, Dataiku, created a Responsible AI e-book that outlines Five Myths — these are measures that many equate with governing AI in an ethical way. I’m sharing those five myths below, as well as my own practical insights and recommendations.
- Myth #1: The Journey to Responsible AI Ends with the Definition of AI Ethics. This is simply not true. Plus, it fails to recognize that ethical AI needs to be balanced with two key objectives: intentionality and accountability. Intentionality ensures that models are designed and behave in ways aligned with their purpose. This includes assurance that data used for AI projects comes from compliant and unbiased sources, plus a collaborative approach to AI projects that ensures multiple checks and balances on potential model bias. Accountability requires centrally controlling, managing, and auditing enterprise AI technology with no shadow IT. Accountability is about having an overall view of which teams are using what data, how, and in which models. Then there’s traceability: if something goes wrong, is it easy to pinpoint where that happened?”
- Myth #2: Responsible AI Challenges Can Be Solved with a Tools-Only Approach. This is a laughable viewpoint that completely discounts the importance of keeping people first. In fact, in my view, AI tools exist solely to support the efficient implementation of the processes and principles defined by the people within a company.
- Myth #3: Problems Only Happen Due to Malice or Incompetence. There’s no denying that putting people first in any technology initiative can introduce risk. This is why having a responsible AI layer built into the business process and systems is necessary.
- Myth #4: AI Regulation Will Have No Impact on Responsible AI. The key point to consider here is how standardized AI regulations will be rolled out and by whom. Will this be through a consortium of companies agreeing on the standards? Will this come through governmental oversight? Companies have been operating under strict compliance and regulatory requirements for decades. This has not slowed progress in any way, but it does have a profound impact on how companies operate, execute strategy, and use technology.
- Myth #5: Responsible AI Is a Problem for AI Specialists Only. The explosion of AI should be a clear indicator that a single person cannot possibly manage how a company approaches ethics and AI. Further, this is not just an “IT thing;” AI is quickly becoming a core technology that impacts all business functions. As such, AI must be understood by the Board, the C-suite, and all decision-makers, not just the technologists.
Explainable AI – What It Is and Why It Matters
“Explainable artificial intelligence (XAI) is a powerful tool for answering how-and-why questions. It is a set of methods and processes that enable humans to comprehend and trust the results and output generated by machine learning algorithms.” This is how H2O.ai, another AI/Hyperautomation Top 10 Short List company, describes Explainable AI.
But I don’t think this description encompasses all of what explainable AI is and should be. H2O.ai has turned this into a tool for companies to utilize, but real explainable AI is much more than a tool. Explainable AI needs to be something that a company practices and implements as a business process and as an accompaniment to Ethical AI.
I would extend the definition above to say explainable AI is a foundational practice incorporated into the fabric of any AI platform (and company) that acts as the “AI provenance,” or record of components, systems, and processes that affect data that’s been collected. It should provide insights for technology teams and business decision-makers. Below, I outline in detail how it can do that for these two core constituencies.
For technology teams, explainable AI should provide visibility into:
- Data sources so teams can know if the sources are trustworthy and whether they are internal or external to a company
- Data usage so IT leaders can know how data is used in the context of a given AI Model, what systems are using the data input and how that influences output, as well as how much data was used to produce the AI output
- Data influence so tech leaders can determine whether certain systems or people influence the data output in a biased way — either intentionally or unintentionally
- How the AI model can be improved not only from a performance perspective but from a quality perspective. Related to that, it should include how and where (internal or external) new AI tools, solutions, or functionality have been developed
- AI/data security so that a company can ensure all data sources and systems are secure, and that cybersecurity teams are up to speed on securing AI tools and output
For business decision-makers and leaders, explainable AI should provide visibility into:
- Competitive AI opportunities to demonstrate that 1) AI is being leveraged to its full potential and 2) how new revenue-generating opportunities can be unlocked to stay competitive and grow
- AI/data compliance in the context of current regulatory requirements and the laws of any country in which a business operates
- AI skills gaps or upskilling opportunities so it can be determined if current people can grow into AI roles or whether new talent is needed today or in the future
- AI security to give a clear indication of how resilient the company is and how it can adapt to “hallucinations” that could influence other systems and create security risks. “AI hallucinations” occur when AI output does not match or is not justified by the training data. This insight will also a give clear indication of whether your company would pass an audit
While this is not a comprehensive outline, it should serve as a starting point to ensure your Explainable AI processes and systems are serving you fully.
Be sure to check out Part 2: Why the rise of Generative AI is increasing urgency to deliver Ethical AI and Explainable AI. Â
Looking for real-world insights into artificial intelligence and hyperautomation? Subscribe to the AI and Hyperautomation channel: