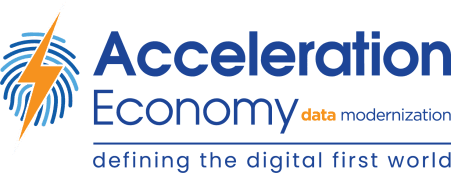
In Part 1 of this series, “How to Treat Deliverables as Assets in AI and Data Projects,” we identified and anticipated the key elements of data projects as well as showed how projects should be treated as assets that must return value. In Part 2, we will discuss how to set up and organize a team of professionals to successfully execute artificial intelligence (AI) and data projects.
Fundamentals – the Why
To successfully execute any project, the right resources are needed; there’s no question about that. So, this is why a team of talented individuals who have experience with AI and data is needed.
Process – the How
When planning for AI, one of the biggest roadblocks leadership faces is finding talent. Nowadays, AI and data disciplines are in high demand. Recruiting people with the right skills is already challenging enough, but even more difficult when, as is often the case, leadership is not well versed in AI or data.
Here are four elements to consider when organizing a team of AI and data professionals.
1. Outsource or in-house? It depends.
Very often, organizations outsource teams via consulting companies and other third parties. This works well if the organization is at the beginning of its data journey.
If the organization has some maturity with its data journey and any kind of data team, then things are different, as in-house resources are available.
2. Diversity is key.
Diversity is not just a human resources buzzword. Team diversity means a team of professionals with diverse educational backgrounds, technical and business expertise, educational levels, and ethnicities.
In data projects, especially in AI development, diversity is a requirement. AI models can be easily biased because, even if trained with “Big Data,” they can only be trained with a limited amount of data in a global and historical context, which is not reflective of the real world — or its situations and people — out there. The most effective way to reduce that bias is by having a diverse team of professionals who can add perspective to the model and interpret the outputs, so that the model can be adjusted accordingly (and often). The last thing any company wants is to create an AI application that favors certain aspects inherently associated with specific groups of individuals within its customer base.
3. Combine diverse knowledge for the win.
To develop a successful data or AI project, it is an absolute must to combine diverse educational backgrounds and knowledge skills. Avoid having an AI project team with only engineers; technical skills need to be blended with business and soft skills. Technical individuals tend to focus too much on technology, programming languages, frameworks, cloud computing, and other related areas in tech. It’s easy for them to lose sight of the original goal, which is why are we doing this project and what value will it add to the organization.
4. Make certain the team leader is balanced.
This is not a trivial point. There are many different leadership styles, and none are wrong. There leaders who are deeply tech-savvy and others with almost no technical background at all. The latter is acceptable, but ultimately technical skills are highly desirable as there are many strategic decisions in which such skills come in handy. On the other hand, a leader who is too in the weeds of technology might fail to see the bigger picture in terms of a project’s ultimate value and utility. Find a team leader who can tow the line between technology and overall project goals. Once they are in place, here are some recommendations for them:
- Keep everybody focused on the goal: add value to the business.
- Don’t let the “technology shine” hypnotize the team.
- Trust the technical people. Not everything is possible even though it may look simple or easy at first sight, and some other things that look impossible are feasible.
While is a lot more to consider in terms of building AI and data project teams, the above comprise the essential elements.
Final Thoughts
There are many different ways to organize a team when a data or AI project is going to be executed; whether it is an external or internal team; whether it is mature or brand-new; and whether it is a CoE (Center of Excellence) or a functional team. To maximize project success, it is essential that the team is diverse in terms of background, individuals, skills, and organizational level. Finally, the team leader must maintain the balance among the team, the project, and the organization. Not an easy task, but very rewarding.
Want more insights into all things data? Visit the Data Modernization channel: