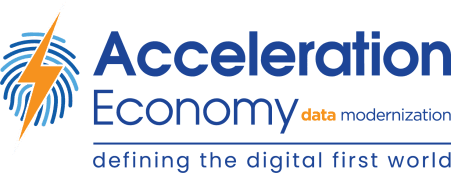
Artificial intelligence (AI) seems to be infused into everything today – cloud services, computer chips, phones, cars, and much more. However, AI is far from perfect and far from sentient. As such, the data output of AI is not always accurate which means that people are still needed to govern, monitor, and make decisions based on imperfect AI systems.
To understand what’s really going on, let’s cover the basics of AI first.
AI Systems and Data Experimentation
Put simply, artificial intelligence is the process by which systems and machines can imitate human cognitive processes. As we all know, the human cognitive process is based on trial and error involving experimentation of events, reaction or feedback, observation of results, and then back to experimentation.
AI systems follow a similar pattern, but the key difference is that systems can only experiment with data, while humans can experiment with data and actions. This is why it is so important to have good data so that the systems can experiment and conduct automated analysis on it.
After the analysis is complete, the systems produce an output based on rules that have been pre-defined by humans who then analyze the data. Reinforcing the need for human intervention and experience to understand WHY the AI generated the specific output, and HOW the output can be used to drive strategic outcomes.
Further, humans are crucial in the process as the AI-generated output can potentially deviate from the original criteria. Depending on the use case of AI, this can have impacts ranging from non-existent to life threatening.
Leveraging The Positives in “Data Drift”
The root cause of AI models going off course is due to something called “data drift”. This occurs when the new input data that the model is processing differs significantly from the original data to which the model was trained. While this may seem like a negative outcome, there are many positive things to consider as they can provide great strategic feedback to the operation team.
One example is the “data drift” may indicate a change in the overall trend of the data and the features collected by the model to produce the results – meaning the whole model pipeline and algorithm used need to be re-evaluated. Additionally, consider looking deeper at the raw data. Perhaps the features that were relevant when the AI model was built are no longer relevant.
Conversely, if there is no change in the trends of the data features, this may point to a data quality, corruption, obsolescence, or governance issues of the latest dataset. Again, this requires having right people monitoring the entire process.
Final Thoughts
Typically, overall data management responsibility falls squarely on the shoulders of the Chief Data Officer and their data teams. That means, in spite of the increase in data volumes and data sources, they are accountable for the data and AI output. Fortunately, many tools are available — both open source and commercially licensed — to optimize MLOps resiliency and manage “data drift” issues.
Finally, CXOs need to ensure they are providing the necessary resources – solutions and people – to the data teams to continuously hone the trustworthiness of the data, which is the foundation for all organizations in our digital-first world.
Bottom line: Both AI-generated and human-generated data needs scrutiny. So, stay alert!
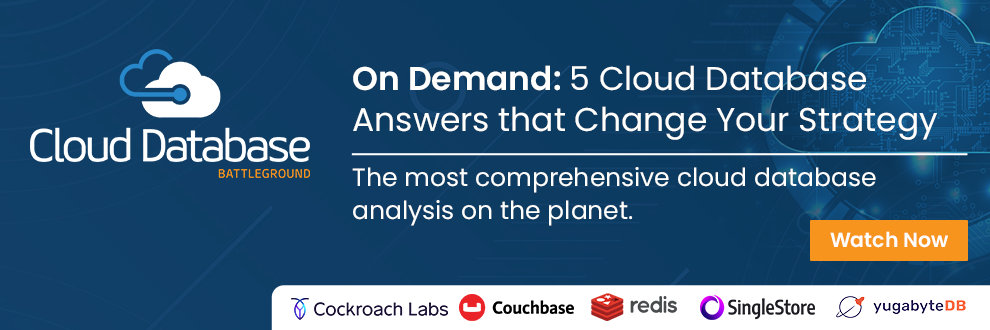