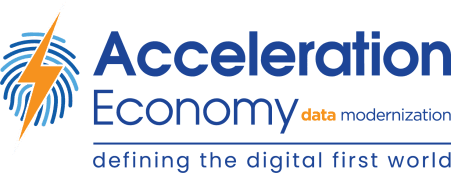
One of the more exciting things happening across a variety of edges is artificial intelligence. It is becoming more prevalent especially on our consumer devices, but also across a fast-expanding range of IoT devices that are enabling intelligent digital twinning of factory equipment or monitoring of crops in a field.
As AI-capable devices and emerging edge infrastructures foster new concepts in distributed AI architectures, business leaders and the C-suite can expect pockets of opportunity to arise across an increasingly cognitive and intelligent edge.
What is Edge AI?
There is a new buzzword in the bustling town of Artificial Intelligence and it is called Edge AI. Like many buzzwords, it is a reference to something that has been going on for a few years now that has brought increasingly powerful AI applications and capabilities to endpoint devices that populate the edge. As mentioned in prior Cutting Edge pieces, the smartphone has played a pivotal and catalytic role in bringing AI to a rapidly expanding universe of devices as well as edge computing infrastructures.
Broadly, Edge AI is interesting in that it brings a new model of computing and intelligence out toward the edge where it didn’t necessarily reside before. This is a big deal that is actively forcing us to evolve our thinking about what is possible with AI through new architectures and technologies that continue to emerge. The impact of Edge AI on distributed system architectures is apparent in many IoT domains industrial and consumer.
A great example of the application of Edge AI is the truly wireless earbud popularized by Apple’s AirPods. These devices are increasingly incorporating advanced AI features such as adaptive noise canceling. Qualcomm, with its Snapdragon Sound, has showcased highly advanced AI-based noise filtering for voice calls that almost entirely eliminates prominent background noise such as the sound of a crumpling bag of chips that might render a conversation near impossible. Incredibly, these AI functions are executed in real-time on some of the most constrained peripherals that we use on a daily basis today.
All of this means that endpoint devices, distributed systems, and applications at the edge are increasing their cognitive, analytic, and intelligent capabilities. Moreover, the learning (model training) aspect of AI-based systems can be designed and deployed away from the hyperscale cloud. This is a big deal. This is why Edge AI is so exciting. It is driving the paradigm and mindset shift for AI computing away from the central cloud.
The Forces Bringing About an Intelligent Edge
Edge AI is making the edge as we knew it and know it more intelligent. It is bringing unprecedented levels of machine cognition, analytics, and intelligence that were typically relegated to the cloud or hefty edge infrastructure in proximity or on-prem. Edge AI is happening due to the following technology forces currently at play:
Neural Processing Units (NPU)
The NPU is a dedicated logic IP or device that is specially designed to execute ML (Machine Learning) and DL (Deep Learning) algorithms with high energy efficiency, especially for devices used in constrained environments. In many ways, they are similar to GPU (Graphic Processing Units) in that they are a class of processors called an accelerator.
Thanks to advances in chip design, integration, and packaging technologies, NPUs are more commonly integrated into microcontrollers that are used in embedded systems, such as your intelligent toaster, industrial PLC, or even a sensor on a medical device.
Moreover, we are seeing more NPUs show up in SoCs (System on Chip) that power our smartphones, smart speakers, smartwatches, and more.
Model Compression
TinyML has become a big thing lately due to its potential to dramatically reduce the size and complexity of ML and DL models that are used in inference operations on extremely power- and compute-constrained devices. Think sensors monitoring well operations in a remote field in the plains of Texas or microclimate conditions in a vineyard in Bordeaux. These scenarios in constrained and rugged environments have always had a demand for fine-grained and continuous condition intelligence.
TinyML uses various techniques of pruning (distilling out redundancies in a neural network) and quantizing a model to reduce its size when it is implemented as an algorithm for inference operations on devices. The trick is compressing your models without losing too much accuracy. TinyML techniques and emerging technologies allow developers to build highly compressed DL models that lose very little of their effectiveness when implemented in the field.
What is the Business Value of AI at the Edge?
At a business level, Edge AI means new ways of looking at automating your business and placing intelligence in your products and services. If you look at Edge AI holistically from a distributed edge system perspective, you can evolve your business functions, processes, and operations toward a zero-touch, self-optimizing modality.
Moreover, Edge AI will help you bring a tighter loop of optimization and learning across your business whether it is in the field or at your corporate headquarters. That means your business is able to leverage AI technologies to enhance the cognition capabilities of your business systems.
Edge AI will also help your organization make sense of unstructured data in a way that fosters new insights from broader corpora of data that were difficult to extract meaning and value out of before. The better news is that the economics of extending AI to the edge is rapidly making sense.
Edge AI can help you improve the security and privacy of your offerings by localizing the execution of inference directly on devices used by your organization as well as your customers. This means fewer data transmitted to, processed, and stored in the cloud.
We are already seeing some of these Privacy First architectures emerge where fingerprint, facial and voice recognition functions are implemented on devices to minimize the exposure of biometric data of the user to third parties, including the service provider and device OEM.
The CXO’s Edge AI imperative
There is no doubt that AI at the edge is changing computing as we know it in so many profound ways. We see it in every release of a new smartphone and with the galaxy of new endpoint devices of all imaginable varieties coming to market on a daily basis. CXOs looking for opportunities to innovate and optimize their business operations across edges will want to get a feel for the kinds of business and consumer applications that are possible with on-device AI.
At the moment, we are seeing the emergence of cognitive edge computing. As you up the maturity of your operational automation, you will want to start thinking about getting on the autonomy maturity curve with Edge AI.
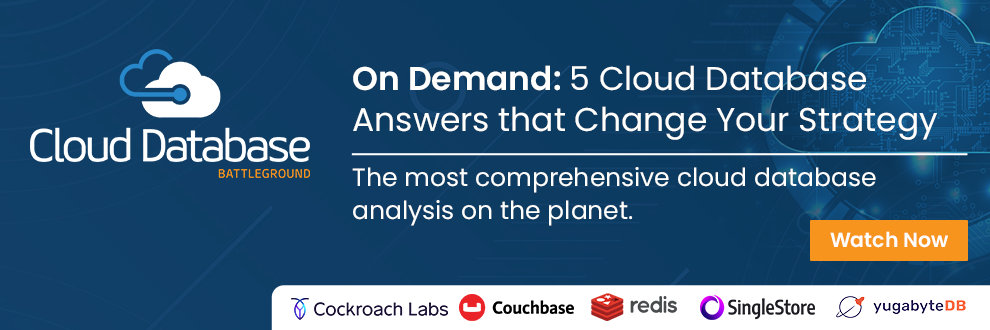